Getting your Trinity Audio player ready...
|
In the race to leverage artificial intelligence, organizations across industries find themselves facing a critical question: Is it better to build AI solutions in-house or to buy them from external vendors? This dilemma is no longer confined to the realm of IT departments and technical specialists. Rather, it has become a company-wide, high-stakes decision that shapes organizational strategy, culture, and future competitiveness.
From predictive analytics for financial institutions to generative language models revolutionizing customer service, AI has demonstrated the potential to streamline processes, unlock new revenue streams, and enhance the employee experience. Yet, as many executives have discovered, the path to a thriving AI ecosystem involves far more than comparing licensing fees or reviewing data security checklists. It demands a behavioral science lens, one that recognizes how deeply AI adoption depends on human attitudes, trust, and willingness to engage with new technologies.
This article offers a research-based, human-centered framework to guide decision-makers through the build-versus-buy debate. It not only tackles the classic concerns—time to market, talent availability, and cost—but also explores the behavioral dynamics that can make or break AI initiatives, from organizational readiness to ethical considerations. Whether your enterprise is embarking on its first AI project or scaling up existing capabilities, these insights can help you make a decision that maximizes long-term value and minimizes cultural friction.
The Rising Stakes of AI Adoption
AI’s potential is visible in both top-line growth and bottom-line efficiency. A study by PwC estimates that AI could deliver additional global economic output of $15.7 trillion by 2030, underscoring the urgency for companies to integrate AI into core operations. At the same time, organizations face mounting pressure to ensure that their AI tools are ethical, transparent, and aligned with evolving regulations.
For many executives, the build-versus-buy dilemma embodies a strategic inflection point. A well-executed AI strategy not only yields competitive advantage but also has the power to transform organizational culture and elevate employee engagement. Conversely, a misaligned approach, where AI feels forced, intrusive, or irrelevant, can erode confidence among both employees and lead to resistance.
Why a Behavioral Science Lens Matters
Most AI adoption frameworks focus on technical feasibility and features. While important, these overlook the behavioral factors that often determine success or failure. Adoption ultimately depends on how people perceive, trust, and use AI in their daily work. Without addressing the cognitive biases and social dynamics that shape behavior, even the most advanced solutions risk rejection. A behavioral science lens helps bridge the gap between technological ambition and human reality, ensuring AI is not just deployed, but actively embraced.
Subscribe to Our Newsletter
Your new guide to the best and latest insights on AI adoption and holistic digital transformation each Friday – from the AdaptAI team.
Key Considerations in the Build vs. Buy Decision
- Speed of Deployment
Buying an AI solution often yields a faster time-to-value. Vendors typically offer tested products, installation support, and pre-built integrations. For organizations seeking immediate productivity gains buying can be compelling. - Strategic Customization
Building AI in-house usually involves custom models tailored to proprietary data, niche customer insights, or unique internal processes. This approach can be slower but may yield sustained competitive differentiation if AI is central to your long-term strategy.
Leaders should assess organizational appetite for change. Employees in a fast-paced environment who are busy spending time on low value tasks may be more receptive to a quick rollout, whereas a culture deeply invested in deliberate and continuous improvement might prefer a gradual build.
- Scarcity of AI Specialists
Data scientists and Machine Learning engineers command premium salaries, and the market remains competitive. Building AI from scratch demands an in-house team capable of handling data preprocessing, model training, and ongoing maintenance.
- Upskilling the Workforce
Training existing staff can mitigate the talent shortfall but requires time, budget, and commitment. Yet, cultivating internal expertise fosters a culture of innovation and self-reliance that may accelerate future AI projects.
Employees often respond favorably to developmental opportunities. Offering them a path to evolve their AI fluency can boost morale. Conversely, bringing in outside talent or vendor teams without involving current staff can trigger perceptions of exclusion.
- Proprietary Data
If your data is highly sensitive, maintaining strict governance may necessitate in-house control. This is particularly relevant under regulations such as HIPAA or GDPR. - Vendor Lock-In
Off-the-shelf AI solutions may rely on proprietary architectures or closed-source models. If you later decide to switch vendors, data portability can be a stumbling block, incurring unexpected costs and operational disruptions.
Trust underpins successful AI adoption. Employees need clarity about who controls the data and how it is used. Overlooking these concerns can erode confidence in both leadership and the technology itself.
- Regulatory Environment
Upcoming frameworks such as the EU AI Act stress auditability and explainability. Whether building or buying, organizations must be equipped to demonstrate compliance across the AI lifecycle. - Bias Mitigation
AI models risk reflecting historical biases embedded in training data. A robust governance framework complete with periodic model reviews and fairness audits, is critical.
Employees are more likely to adopt AI when they perceive it as fair and transparent. A “black box” approach not only invites skepticism but can also provoke ethical dilemmas for those directly impacted by AI-driven decisions.
- Change Management
AI often reshapes how tasks are performed. Without a deliberate strategy to manage this transition with clear communication and training, resistance can stall or derail adoption. - Alignment with Workflows
AI tools that integrate smoothly into existing processes are more likely to see more enthusiastic uptake. If deployment introduces complex new steps or unclear role definitions, employees may revert to familiar routines.
- Upfront vs. Ongoing Costs
Building AI incurs high initial expenses. For example, computing infrastructure and software, while buying involves licensing fees and potentially usage-based costs. Over time, either model may prove more cost-efficient depending on scale and complexity of your organization’s needs. - Scalability
In-house AI that is deeply integrated with core systems may eventually scale more economically, whereas vendor solutions might incur incremental fees or offer limited customization as needs evolve.
Making the Decision: A Comprehensive Roadmap
Below is a five-step roadmap that unifies these considerations into a structured approach. While every organization’s context is unique, these steps offer a behavioral science-informed scaffold to guide reflection and decision-making.
Clarify the Strategic Vision
- Identify Core Objectives: Is AI essential to achieving a distinctive competitive advantage, or is it primarily an operational enabler?
- Anticipate Future Needs: Project how AI might evolve in your sector, keeping an eye on emerging technologies.
Conduct an Organizational Readiness Assessment
- Evaluate Current Skills: Determine whether your workforce can sustain an in-house AI program and where targeted upskilling may be needed.
- Gauge Cultural Receptivity: Use employee surveys or focus groups to assess existing attitudes, fears, and sources of excitement surrounding AI.
→ Get free access to our AI Readiness Assessment today. Evaluate your organization’s technical capabilities, workforce alignment, and cultural preparedness for AI adoption. Start making informed, strategic AI decisions now. Request access.
Develop a Governance and Ethics Framework
- Set Oversight Mechanisms: Establish roles, committees, and processes for accountability and risk management, regardless of whether you build or buy.
- Plan for Transparency: Define how you will explain AI decisions and address bias, which are critical for building trust internally and externally.
Run a Cost-Benefit and Timeline Analysis
- Compare Immediate vs. Long-Term ROI: Weigh the speed of buying against the strategic advantage of building. Factor in hidden costs such as vendor lock-in and compliance overhead.
- Phased Approach: When feasible, pilot a smaller use case to validate assumptions, refine models, and build early success stories.
Execute with Change Management in Mind
- Engage Employees Early: Involve key stakeholders in scoping, vendor selection, or prototyping. Leverage early champions to demonstrate AI’s value.
- Maintain Iterative Feedback Loops: Encourage continuous user feedback, refine processes, and track metrics on both technical performance and employee sentiment.
Through this systematic approach, leaders can integrate behavioral insights into each stage of the decision, helping ensure the chosen path gains real traction among the people who matter most.
Want personalized guidance on applying this framework to your organization’s unique challenges?
Reimagining AI Adoption as a Human-Centric Strategy
In debates about whether to build or buy AI, it’s easy for leaders to focus on product features, budget, and timelines. Yet the success of any AI initiative depends fundamentally on human elements, the chief of which are the employees’ willingness to learn and adapt.
By applying a behavioral science perspective, organizations can transcend a one-dimensional view of AI adoption and instead orchestrate a transformation that fosters genuine engagement. Whether you opt to purchase an AI platform for speedy deployment or invest in building proprietary models for strategic advantage, the key to sustained impact lies in preparing your people to embrace a new era of data-driven decision-making.
Authored by:
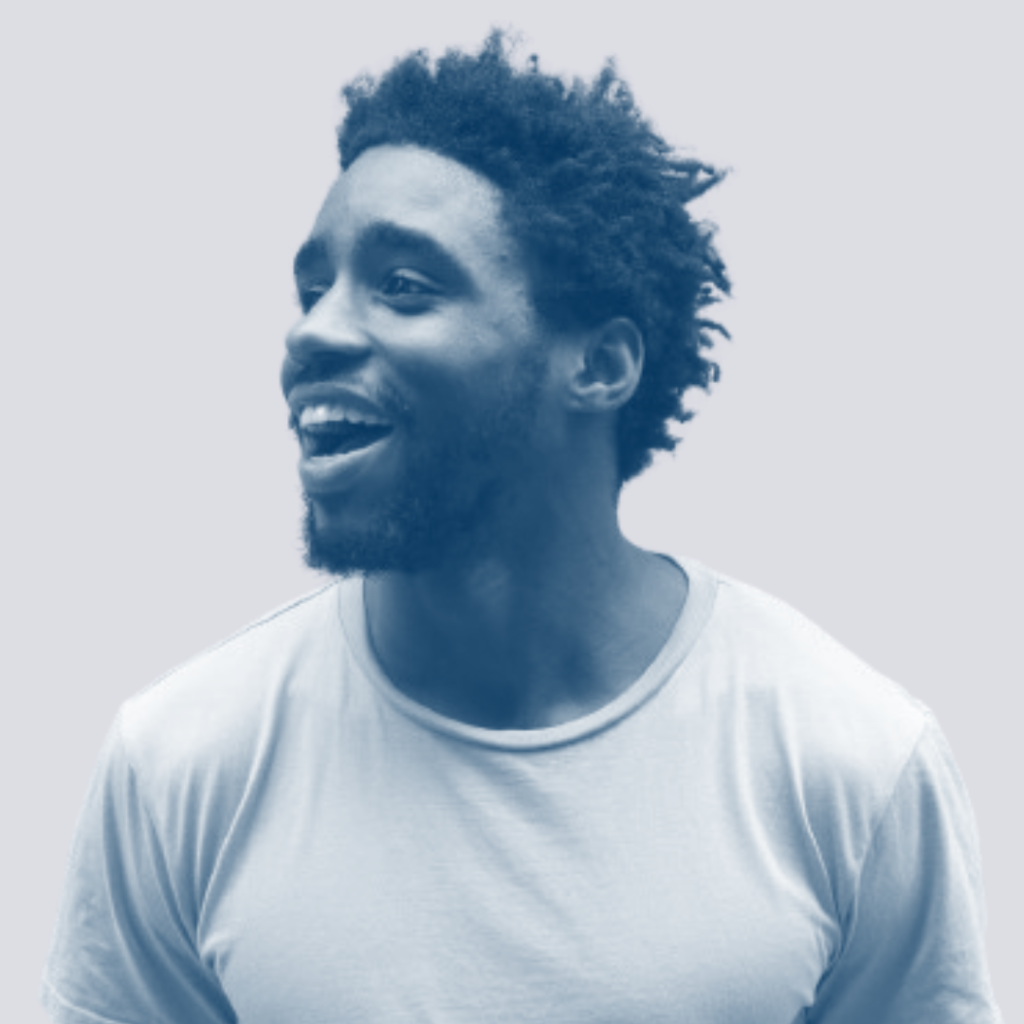