Getting your Trinity Audio player ready...
|
It’s no secret AI is the hottest buzzword around the metaphorical water cooler. Whether you’re excited or filled with dread, there’s no denying AI has potential to shift the world of work as we know it. From detecting tumors in healthcare with unprecedented accuracy to improving customer service and advancing agricultural techniques, AI can fundamentally reshape how industries operate and the value bring the world.
Worldwide spending on artificial intelligence, including AI-enabled applications, infrastructure, and related services, is forecasted to reach $632 billion by 2028, more than doubling from current levels. Generative AI alone is expected to account for $202 billion, or 32% of that spending. Despite these figures, many businesses are struggling to see returns on their AI investments.
So where is it going wrong? Our research points to three critical areas that stand in the way of effective AI adoption, and we’ll explain what you can do at your organization to overcome them.
Lack of Strategic Focus
Many AI projects fail because they lack a clear purpose. Organizations are blindly jumping into deploying tools as a reactionary response without fully understanding how these initiatives align with broader business goals. The result? Misallocated resources, poorly vetted vendors, and a lack of measurable success.
Common Mistakes
- Isolated AI Initiatives: This lack of holistic planning leads to fragmented solutions that don’t add meaningful, sustainable value across the organization.
- Misalignment with Business Goals: Organizations fail to align AI initiatives with critical, present, and expensive business priorities, resulting in solutions that may be technically impressive but fail to address key challenges the business faces or generate significant ROI.
- Chasing Trends Without Preparation: Many companies adopt AI in response to market or competitor signaling, without fully evaluating their organizational readiness or understanding what success looks like. This trend-chasing leads to a disengaged workforce as well as a host of unmet expectations.
Is Your Organization AI-Ready?
Our AI readiness assessment can help you understand your readiness level across key pillars and how you can improve your business. The demo version is free of charge – feel free to reach out and get access.
How Leaders Can Overcome This
Strategic AI Roadmap: The key to overcoming these barriers is developing a well-defined AI strategy that is tightly aligned with the organization’s overall, and articulated business objectives. By focusing on high-impact use cases, organizations can avoid spreading resources too thin and ensure that AI efforts contribute to long-term goals.
Cross-functional Collaboration: Breaking down silos is essential for building a cohesive AI strategy. AI is not just a technical initiative; it requires close collaboration between business units, data and technology professionals, and leadership to ensure that solutions are designed with a comprehensive understanding of the business landscape. Establishing cross-functional teams ensures that AI projects are guided by technical expertise, business acumen, and most importantly, organizational culture is the surest road to sustainable success. This collaborative approach also helps maintain alignment between AI initiatives and evolving business goals.
Start with Focused, Practical Projects: Instead of launching large-scale, complex AI projects from the outset, organizations should begin with smaller, well-defined initiatives that directly address specific business challenges. These early projects can serve as “quick wins” that build internal confidence and demonstrate the tangible benefits of AI. As these initial successes are realized, they can serve as a foundation for scaling AI efforts more broadly, with lessons learned from early initiatives informing larger, more complex transformations.
With over 200 brands operating in more than 180 countries, Procter & Gamble (P&G) needed a smarter way to predict demand and manage inventory. Rather than blindly adopting the latest AI tools, they took a step back and asked, “How can this technology actually move the needle for our business goals?” From there, they developed an AI-powered supply chain control tower that integrates data from sources like sales trends, weather patterns, and production schedules to make real-time adjustments.
The results were pretty ground-braking, beyond cost savings, they improved product availability that directly benefited their customers. It’s a powerful reminder of what AI can do when it is approached with a clear purpose and aligned with business priorities.

Data Complexity and Quality
The elephant in the room—and often hidden under the carpet—is the state of data in most organizations. AI relies heavily on high-quality data to function effectively. However, silos, incomplete data, inaccuracies, and a lack of data centralization prevent AI from delivering reliable insights. Without a fluid, integrated data system, these tools risk generating poor or even harmful results, perpetuating bias, and missing the mark on predictions.
Key Challenges
- Data Silos: One of the most persistent challenges is the presence of disparate data sources that are not interconnected. Different departments or systems store valuable data in isolation, preventing a holistic view that algorithms are best served with. These silos not only make it difficult to leverage data effectively, but they also limit cross-functional insights that could drive better decision-making. For AI to work optimally, these silos need to be broken down, ensuring seamless data sharing across systems and departments.
- Poor Data Quality: Inaccurate, incomplete, or inconsistent data can severely undermine the performance of these models. AI is only as good as the data it is trained on; if that data is flawed, outputs too will be unreliable. Poor data quality can lead to skewed insights and perpetuate bias. Ensuring that data is accurate, validated, and up-to-date is crucial for reliable AI deployment.
- Data Governance: Many organizations lack a clear structure or policies for managing their data. Without a well-defined governance framework, companies struggle with data ownership, security, compliance, and accountability. As AI becomes increasingly integrated into decision-making processes, data governance becomes essential in ensuring ethical, secure, and effective use of data.
Subscribe to AI Pulse
Your new guide to the best and latest insights on AI adoption and holistic digital transformation each Friday – from the AdaptAI team.
How Leaders Can Overcome This
Data Management Strategy: Developing a robust data management framework is crucial for any AI initiative. Organizations need standardized data processes, clear governance policies, and advanced tools to handle the complexity of vast, multi-source data sets. A well-defined data management strategy helps ensure data is accurate, accessible, and consistent across the enterprise.
Data Integration: Integration is key to breaking down data silos and creating a unified data repository. Leaders should prioritize investing in tools and processes that allow for seamless data integration across different systems and departments. A consolidated view of data ensures that AI models work with comprehensive and reliable information, leading to more accurate predictions and insights.
Building a Data-Centric Culture: Beyond infrastructure and governance, leaders should cultivate a data-centric culture where data quality and governance are embedded into the organization’s daily operations. Investing in the right tools and training employees to prioritize data quality can drive long-term improvements, ensuring that AI projects start with the right foundation.
Did you know that Northwestern Mutual, a financial services giant, found itself held back by the villain of siloed legacy data? Without a unified process and approach, they couldn’t fully deliver on their promise of personalized, seamless customer experiences. They decided then to consolidate their data into a centralized platform. This wasn’t just about cleaning up their systems, it was about creating a foundation for transformation. By ensuring their data was accurate, integrated, and accessible, they could finally unleash AI models capable of delivering precise risk assessments and tailored financial advice.
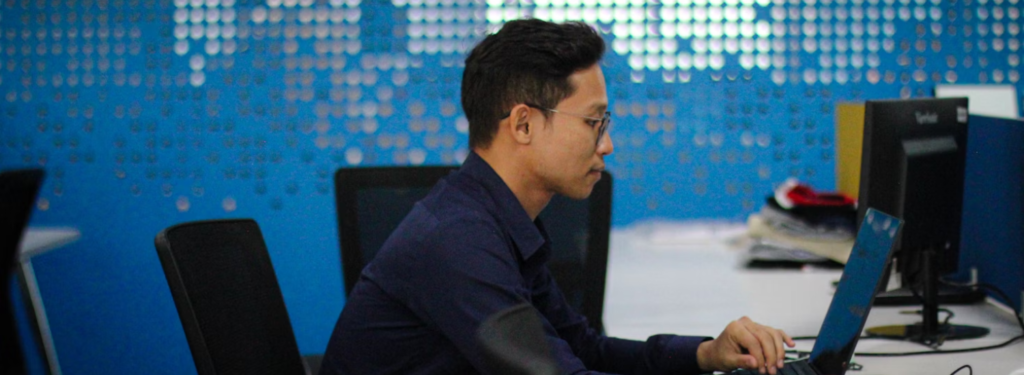
Cultural Resistance and Fear
Perhaps the most insidious challenges are the human factors related to effective AI adoption. Employees often fear that AI will replace their jobs or feel that it’s a distraction from more pressing business issues. These concerns, especially when left unaddressed, can create a deep reluctance to embrace AI solutions. Fear of the unknown, combined with skepticism about how AI might impact roles, often leads to hesitation or outright opposition.
Key Challenges
- Fear of Job Displacement: The anxiety surrounding automation and AI replacing human labor is prevalent. Many employees view AI as a tool designed to reduce workforce size rather than enhance productivity, which leads to a sense of vulnerability.
- Lack of AI Literacy: A significant knowledge gap exists when it comes to understanding AI’s true capabilities and limitations. Without sufficient AI literacy, employees may jump to conclusions about the risks, focusing on potential negative outcomes instead of the opportunities AI presents for improving their roles.
- Change Resistance: People generally prefer stability and familiarity in their work environment. Introducing new, disruptive technologies like AI may require employees to step outside their comfort zones and adapt to new ways of working, which can provoke resistance or even pushback.
How Leaders Can Overcome This
Change Management and Transparent Communication: Effective change management begins with leadership actively addressing employees’ concerns through clear and ongoing communication. It’s crucial to explain how AI is not designed to replace human workers but rather to augment their efforts. Highlighting specific examples of how AI will automate routine tasks and free up time for more meaningful, creative work can help diminish fears of obsolescence. Transparency is key—leaders must clarify the organization’s vision for AI and demonstrate its role in achieving broader business goals.
Training and Upskilling Initiatives: To alleviate fears and build confidence, organizations should invest in upskilling their workforce. Offering AI training programs that equip employees with new skills not only quells anxiety but also empowers them to take on AI-enhanced roles. By positioning AI as a tool for human advancement rather than replacement, companies can foster a culture of continuous learning and innovation.
Create AI Champions: A powerful way to overcome resistance is by identifying and cultivating internal “AI champions.” These individuals, who are well-versed in AI and understand its benefits, can serve as advocates for change within the organization. By showcasing real-life examples of how AI has improved efficiency, innovation, and job satisfaction, these champions can help demystify AI and build trust among their peers. Peer-to-peer advocacy is often more persuasive than top-down mandates, as employees are more likely to trust colleagues who share their concerns.
Measurement-forward Approach to Adoption: Finally, adopting an evidence-based approach to AI implementation can ease fears. Leaders should focus on small, incremental changes where AI demonstrates clear measurable benefits. This phased rollout allows employees to gradually experience AI’s positive impact firsthand, reducing apprehension and building acceptance over time. By presenting data and case studies that show how AI can streamline processes and create opportunities for human workers, leaders can transform fear into enthusiasm for innovation.
Lead your team towards success!
Our in-depth sessions and people-oriented interventions are designed to ensure a swift AI adoption across your enterprise.
Conclusion
AI has the potential to revolutionize industries, but businesses must first address the most critical barriers to its adoption: lack of strategic focus, data complexity, and cultural resistance. Leaders are pivotal in navigating these challenges by aligning AI initiatives with business goals, ensuring data readiness, and cultivating a culture of innovation.
To unlock AI’s full potential, businesses must develop a holistic approach to AI adoption. This means not only refining their AI strategies but also ensuring data readiness and cultivating norms and behaviors that embrace technological change. By doing so, organizations can position themselves to harness the transformative power of AI and drive sustainable, long-term success.
Now is the time for organizations to act—by addressing these barriers head-on, they can pave the way for AI to become a true driver of innovation and competitive advantage.